15 Apr 2019
Slowly but surely, the Animation Compression Library has now reached v1.2 along with an updated v0.3 Unreal Engine 4 plugin. The most notable changes in this release are as follow:
- More compilers and architectures added to continuous integration
- Accuracy bug fixes
- Floating point sample rate support
- Dramatically faster compression through the introduction of a compression level setting
TL;DR: Compared to UE 4.19.2, the ACL plugin compresses up to 1.7x smaller, is up to 3x more accurate, up to 158x faster to compress, and up to 7.5x faster to decompress (results may vary depending on the platform and data).
Note that UE 4.21 introduced changes that significantly sped up the compression with its Automatic Compression codec but I haven’t had the time to setup a new branch with it to measure.
UE 4 plugin support and progress
Now that ACL properly supports a floating point sample rate, the UE4 plugin has reached feature parity with the stock codecs.
As announced at the GDC 2019, work is ongoing to refactor the Unreal Engine to natively support animation compression plugins and is currently on track to land with UE 4.23. Once it does, the plugin will be updated once more, finally reaching v1.0 on the Unreal marketplace for free.
Lighting fast compression
One of the most common feedback I received from those that use ACL in the wild (both within UE4 and outside) was the desire for faster compression. The optimization algorithm is very aggressive and despite its impressive performance overall (as highlighted in prior releases), some clips with deep bone hierarchies could take a very long time to compress, prohibitively so.
In order to address this, a new compression level was introduced in the compression settings to better control how much time should be spent attempting to find an optimal bit rate. Higher levels take more time but yield a lower memory footprint. A total of five levels were introduced but the lowest three currently behave the same for now: Lowest, Low, Medium, High, Highest. The Highest level corresponds to what prior releases did by default. After carefully reviewing the impact of each level, a decision was made to make the default level be Medium instead. This translates in dramatically faster compression, identical accuracy, with a very small and acceptable increase in memory footprint. This should provide for a much better experience for animators during production. Once the game is ready to be released, the animations can easily and safely be recompressed with the Highest setting in order to squeeze out every byte.
In order to extract the following results, I compressed the Carnegie-Mellon University motion capture database, Paragon, and Fortnite in parallel with 4 threads using ACL standalone. Numbers in parenthesis represent the delta again Highest.
Compressed Size |
Highest |
High |
Medium |
CMU |
67.05 MB |
68.85 MB (+2.7%) |
71.01 MB (+5.9%) |
Paragon |
206.87 MB |
211.81 MB (+2.4%) |
218.58 MB (+5.7%) |
Fortnite |
491.79 MB |
497.60 MB (+1.2%) |
507.11 MB (+3.1%) |
Compression Time |
Highest |
High |
Medium |
CMU |
24m 57.59s |
11m 51.48s |
6m 20.89s |
Paragon |
4h 55m 42.57s |
1h 19m 36.01s |
29m 21.65s |
Fortnite |
8h 13m 1.66s |
2h 29m 59.37s |
1h 3m 18.17s |
Compression Speed |
Highest |
High |
Medium |
CMU |
977.36 KB/sec |
2057.24 KB/sec (+2.1x) |
3842.79 KB/sec (+3.9x) |
Paragon |
246.79 KB/sec |
916.82 KB/sec (+3.7x) |
2485.58 KB/sec (+10.1x) |
Fortnite |
613.56 KB/sec |
2016.82 KB/sec (+3.3x) |
4778.65 KB/sec (+7.8x) |
And here are the default settings in action on the animations from Paragon with the ACL plugin inside UE4:
|
ACL Plugin v0.3.0 |
ACL Plugin v0.2.0 |
UE v4.19.2 |
Compressed size |
234.76 MB |
226.09 MB |
392.97 MB |
Compression ratio |
18.22 : 1 |
18.91 : 1 |
10.88 : 1 |
Compression time |
30m 14.69s |
6h 4m 18.21s |
15h 10m 23.56s |
Compression speed |
2412.94 KB/sec |
200.32 KB/sec |
80.16 KB/sec |
Max ACL error |
0.8623 cm |
0.8590 cm |
0.8619 cm |
Max UE4 error |
0.8601 cm |
0.8566 cm |
0.6424 cm |
ACL Error 99th percentile |
0.0094 cm |
0.0116 cm |
0.0328 cm |
Samples below ACL error threshold |
99.19 % |
98.85 % |
84.88 % |
The 99th percentile and the number of samples below the 0.01 cm error threshold are calculated by measuring the error of every bone at every sample in each of the 6558 animation clips. More details on how the error is measured can be found here.
In this new release, the decompression performance remains largely unchanged. It is worth noting that a month ago my Google Nexus 5X died abruptly and as such performance numbers will no longer be tracked on it. Instead, my new Google Pixel 3 will be used from here on out.
What’s next
The next release v1.3 currently scheduled for the Fall 2019 will aim to tackle commonly requested features:
- Faster decompression in long clips by optimizing seeking
- Multiple root transform support (e.g. rigid body simulation compression)
- Scalar track support (e.g. float curves for blend shapes)
- Faster compression in part by using multiple threads to compress a single clip (which will help the UE4 plugin a lot)
If you use ACL and would like to help prioritize the work I do, feel free to reach out and provide feedback or requests!
25 Jan 2019
New year, new stats! A few months ago, Epic agreed to let me use their Fortnite animations for my open source research with the Animation Compression Library (ACL). Following months of work to refactor Unreal Engine 4 in order to natively support animation compression plugins, it has finally entered the review stage on Epic’s end. While I had hoped the changes could make it in time for Unreal Engine 4.22, due to unforeseen delays, 4.23 seems a much more likely candidate.
Even though the code isn’t public yet, the new updated ACL plugin kicks ass and Fortnite is a great title to showcase it with. The real game uses the classic UE4 codecs but I recompressed everything with the latest and greatest. After spending several hundred hours compressing the animations, fixing bugs, and iterating I can finally present the results.
TL;DR: Inside Fortnite, ACL shines bright with 2x faster compression, 2x smaller memory footprint, and higher accuracy. Decompression is 1.6x faster on desktop, 2.3x faster on a Samsung S8, and 1.2x faster on the Xbox One.
Methodology
For the UE4 measurements I used a modified UE 4.21 with its default Automatic Compression. It tries a list of codecs in parallel and selects the optimal result by considering both size and accuracy.
ACL uses a modified version of the open source ACL Plugin v0.2.2. It uses its own default compression settings and in the rare event where the error is above 1cm, it falls back automatically to safer settings.
Although the UE4 refactor doesn’t change the legacy codecs, it does speed up their decompression a bit compared to previous UE4 releases. That is one of many benefits everyone will get to enjoy as a result of my refactor work regardless of which codec is used.
Error measurements
While the UE4 and ACL error measurements never exactly matched, they historically have been very close for every single clip I had tried, until Fortnite. As it turns out, some exotic animations brought to light the fact that some subtle differences in how they both measure the error can lead to some large perceived discrepancies. This has now been documented in the plugin here.
Three differences stand out: how the error is measured, where the error is measured in space, and where the error is measured in time. You can follow the link above for the gory details but the jist is that ACL is more conservative and more accurate in how it measures the error and it should always be trusted over what UE4 reports in case of doubt or disagreement.
It is worth noting that because ACL does not currently support a floating point sample rate (e.g 28.3 FPS), those clips (and there are many) have a higher reported error with UE4 because by rounding, we are effectively time stretching those clips a tiny bit. They still look just as good though. This will be fixed in the next version.
The animations
I extracted all the non-additive animations regardless of whether they were used by the game or not: a grand total of 8304 clips! A total raw size of 17 GB and roughly 17.5 hours worth of playback.
Fortnite has a surprising number of exotic clips. Some take hours to compress with UE4 and others have a range of motion as wide as the distance between the earth and the moon! These allowed me to identify a number of very subtle bugs in ACL and to fix them.
Compression stats
|
ACL Plugin |
UE4 |
Compressed size |
498.21 MB |
1011.84 MB |
Compression ratio |
35.55 : 1 |
17.50 : 1 |
Compression time |
12h 38m 04.99s |
23h 8m 58.94s |
Compression speed |
398.72 KB/sec |
217.62 KB/sec |
Max ACL error |
0.9565 cm |
8392339 cm |
Max UE4 error |
108904.6797 cm |
8397727 cm |
ACL Error 99th percentile |
0.0309 cm |
2.1856 cm |
Samples below ACL error threshold |
97.71 % |
77.37 % |
Once again, ACL performs admirably: the compression speed is twice as fast (1.83x), the memory footprint reduces in half (2.03x smaller), and the accuracy is right where we want it. This is also in line with the previous results from Paragon.
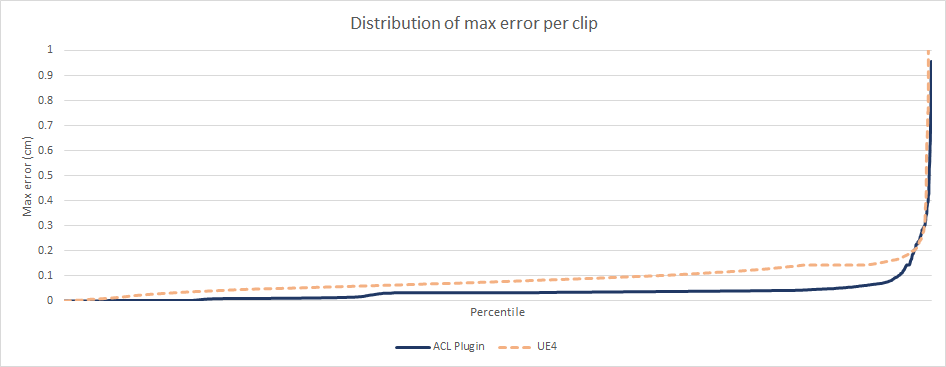
UE4’s accuracy struggles a bit with a few clips but in practice the error might not be visible as the overwhelming majority of samples are very accurate. This is consistent as well with previous results.
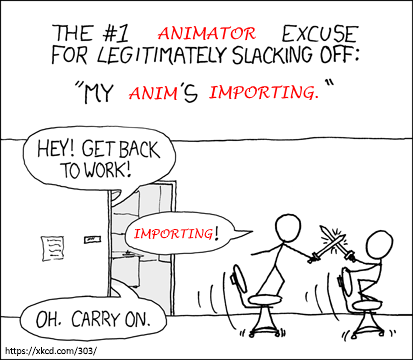
A handful of clips contribute to a large portion of the UE4 compression time and its high error. One clip in particular stands out: it has 1167 bones, 8371 samples at 120 FPS, and a total raw size of 372.66 MB. Its range of motion peaks at 477000 kilometers away from the origin! It truly pushes the codecs to their absolute limits.
|
ACL Plugin |
UE4 |
Compressed size |
71.53 MB |
220.87 MB |
Compression ratio |
5.21 : 1 |
1.69 : 1 |
Compression time |
1m 38.07s |
4h 51m 59.13s |
Compression speed |
3891.19 KB/sec |
21.78 KB/sec |
Max ACL error |
0.0625 cm |
8392339 cm |
Max UE4 error |
108904.6797 cm |
8397727 cm |
It takes almost 5 hours to compress with UE4! In comparison, ACL zips through in well under 2 minutes. While it tries its best with the default settings it ultimately ends up using the safety fallback and thus compresses twice in that amount of time.
Overall, if you added the ACL codec to the Automatic Compression list, here is how it would perform:
- ACL is smaller for 7711 clips (92.86 %)
- ACL is more accurate for 7576 clips (91.23 %)
- ACL has faster compression for 5704 clips (68.69 %)
- ACL is smaller, better, and faster for 5017 clips (60.42 %)
- ACL wins Automatic Compression for 7863 clips (94.69 %)
Decompression stats
Fortnite has the handy ability to create replays. These make gathering deterministic profiling numbers a breeze. The numbers that follow are from a 50 vs 50 replay. On each platform, a section of the replay with some high intensity action was profiled.
Desktop
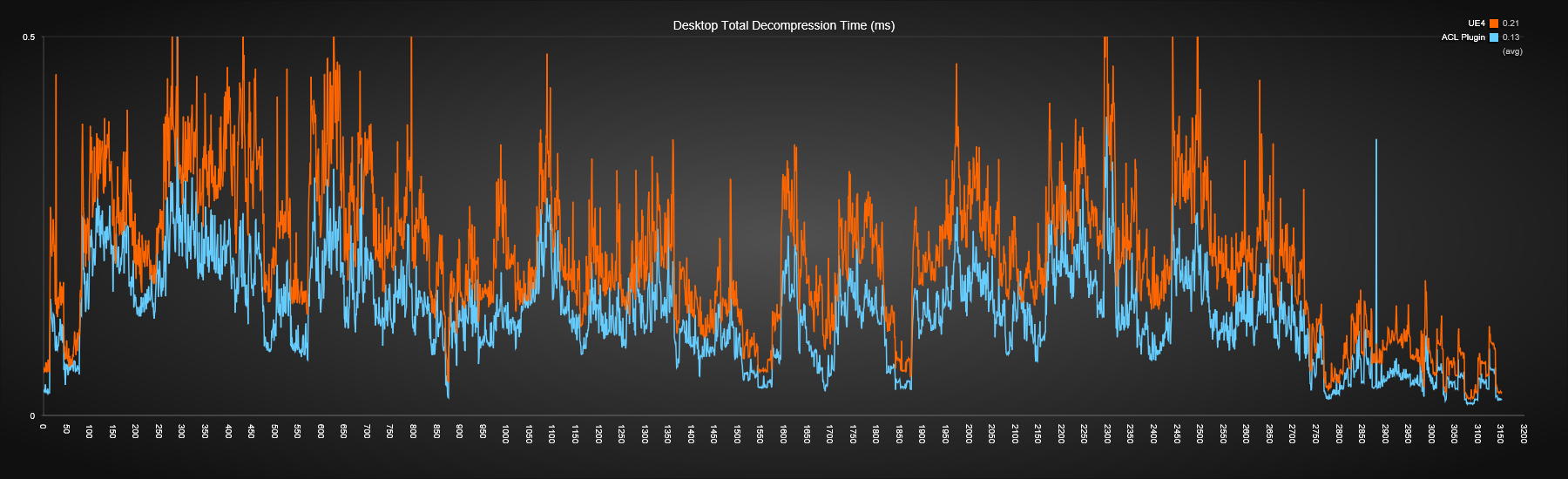
The performance on desktop looks pretty good. ACL is consistently faster, about 38% on average. It also appears a bit less noisy, a direct benefit of the improved cache friendliness of its algorithm.
Samsung S8
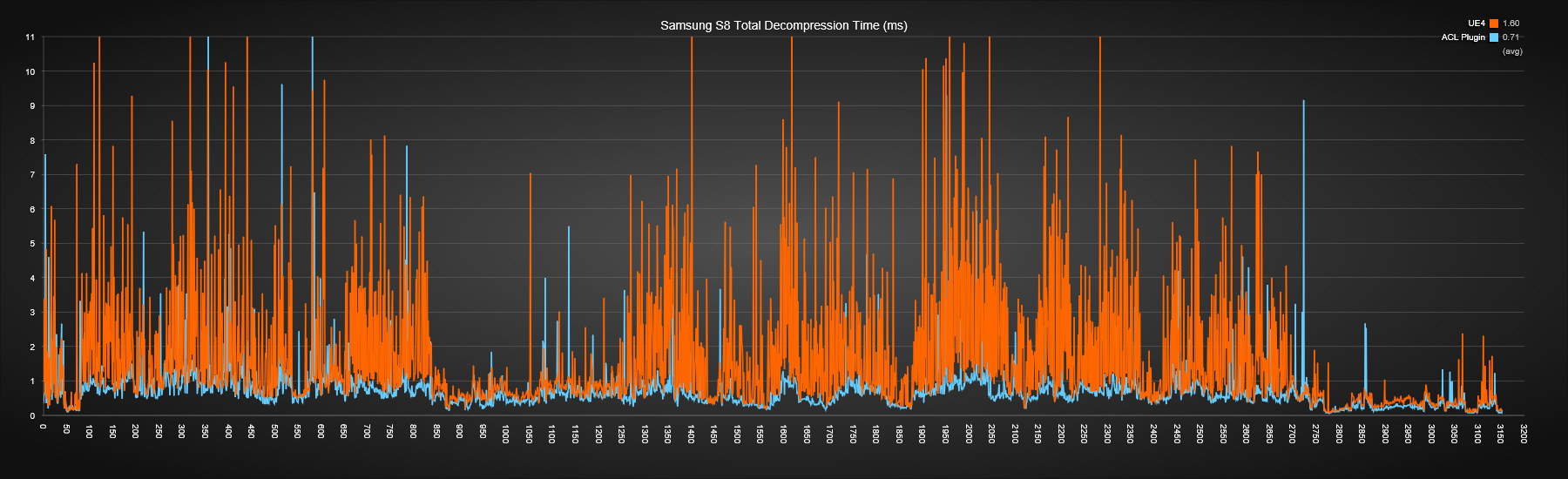
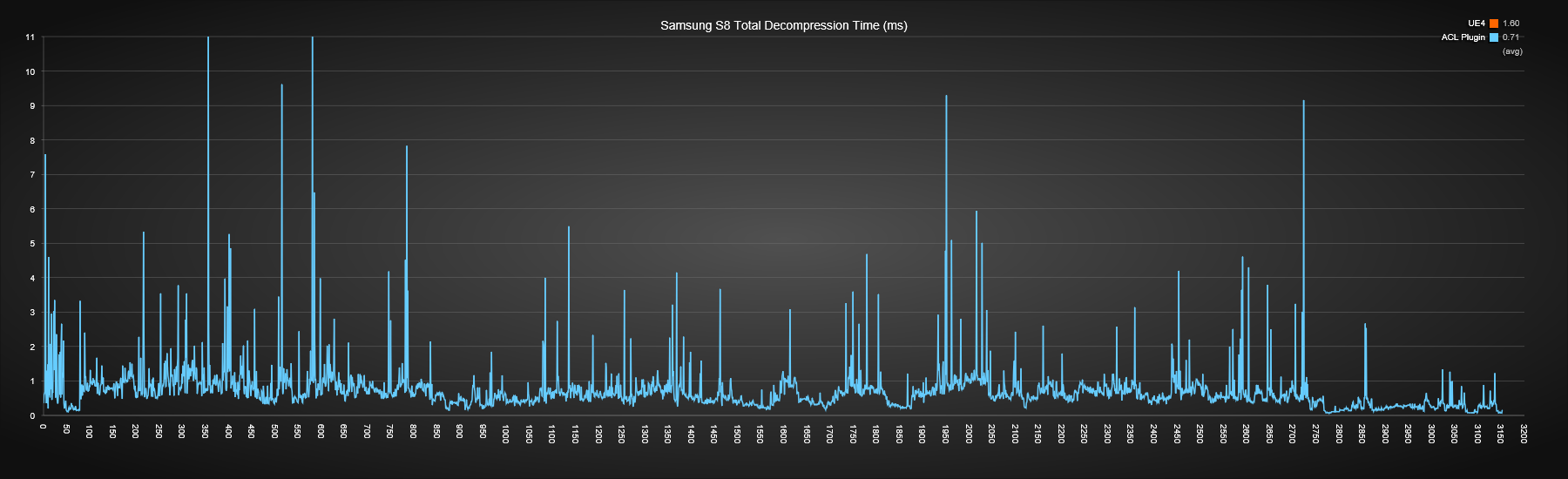
ACL really shines on mobile. On average it is 56% faster but that is only part of the story. On the S8 it appears that the core is hyperthreaded and another thread does heavy work and applies cache pressure. This causes all sorts of spikes with UE4 but in comparison, the cache aware ACL allows it to maintain a clean and consistent performance.
Hyperthreading on the CPU (and the GPU) works, roughly speaking, by the processor switching to another thread already executing when it notices that the current thread is stalled waiting on a slow operation, typically when memory needs to be pulled into the cache. Both threads are executing in the sense that they have data being held in registers but only one of them advances at a time on that core. When one stalls, the other executes.
When you have a piece of code that triggers a lot of cache misses, such as some of the legacy UE4 codecs, the processor will be more likely to switch to the other hyperthread. When this happens, execution is suspended and it will only resume once the other thread stalls or the time slice expires. This could be a long time especially if the other hyperthread is executing cache friendly code and doesn’t otherwise stall often.
This translates into the type of graph above where there is heavy fluctuation as the execution time varies widely from the noise of the neighbor hyperthread.
On the other hand, when the code is cache friendly, it doesn’t give the chance to the other thread to run. This gives a nice and smooth graph for that current thread as the risk of long interruptions is reduced. When the code is that optimized, hyperthreading typically doesn’t help speed things up much as both threads compete for the same time slice with few opportunities to hide stalling latency. This is also what I observed when measuring the compression performance. In theory due to the higher cache pressure, performance could even degrade with hyperthreading but in practice I haven’t observed it, not with ACL at least.
Xbox One
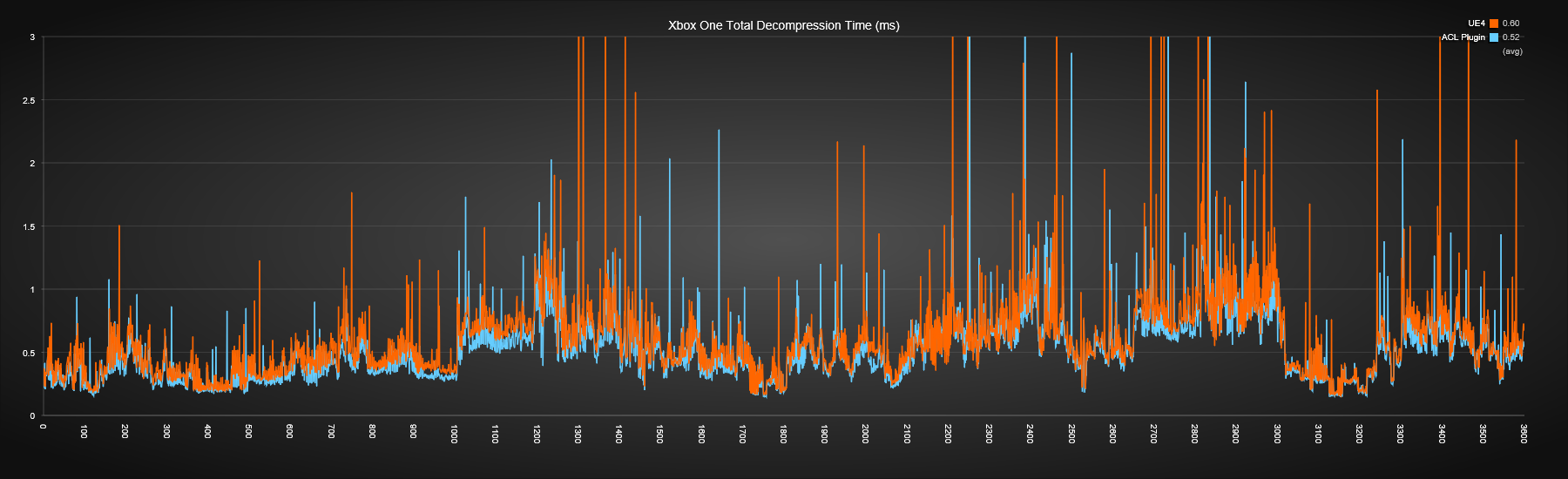
On the Xbox One ACL is about 13% faster on average. Both lines seem to have very similar shapes unlike the previous two platforms due in large part to the absence of hyperthreading. There are a few possibilities as to why the gain isn’t as significant on this platform:
- The MSVC compiler does not generate assembly that is as clean as it generates on PC, it’s certainly sub-optimal on a few points. It fails to inline many trivial functions and it leaves around unnecessary shuffle instructions.
- Perhaps the other threads that share the L2 thrash the hardware prefetcher, preventing it from kicking in. ACL benefits heavily from hardware prefetching.
- The CPU is quite slow compared to the speed of its memory. This reduces the benefit of cache locality as it keeps L2 cache misses fairly cheap in comparison.
The last two points seems the most likely culprits. ACL does a bit more work per sample decompressed than UE4 but everything is cache friendly. This gives it a massive edge when memory is slow compared to the CPU clock as is the case on my desktop, the Samsung S8, and lots of other platforms.
Conclusion
With faster compression, faster decompression on every platform, a smaller memory footprint, and higher accuracy, ACL continues to shine in UE4 and it won’t be long now before everyone can find it on the marketplace for free.
In the meantime, in the next few months I will perform another release of ACL and its plugin with all the latest fixes made possible with Fortnite’s data.
Status update
To my knowledge, the first game released with ACL came out in November 2018 with the public UE4 plugin: OVERKILL’s The Walking Dead. I was told it reduced their animation memory footprint by over 50% helping them fit within their console budgets.
A number of people have also integrated it into their own custom game engines and although I have no idea if they are using it or not, Remedy Entertainment has forked ACL!
Last but not least, I’d like to extend a special shout-out to Epic for allowing me to do this and to the ACL contributors!
19 Jan 2019
Almost two years ago now, I began writing the Animation Compression Library. I set out to build it to be production quality which meant I needed a whole lot of optimized math. At the time, I took a look at the landscape of math libraries and I opted to roll out my own. It has served me well, propelling ACL to success with some of the fastest compression and decompression performance in the industry. I am now proud to announce that the code has been refactored out into its own open source library: Realtime Math v1.0 (RTM) (MIT license).
There were a few reasons that motivated the choice to move the code out on its own:
- A significant amount of the ACL Continuous Integration build time is compiling and running the math unit tests which slows things down a bit more than I’d like
- It decouples code that will benefit from being on its own
- I believe it has its place in the landscape of math libraries out there
In order to support that last point, I reviewed 9 other popular and usable math libraries for realtime applications. I looked at these with the lenses of my own needs and experience, your mileage may vary.
Disclaimer: the list of reviewed libraries is in no way exhaustive but I believe it is representative. Note that Unreal Engine 4 is included for informational purposes as it isn’t really usable on its own. Libraries are listed in no particular order and I tried to be as objective as possible. If you spot any inaccuracies, don’t hesitate to reach out.
The list: Realtime Math, MathFu, vectorial, VectorialPlusPlus, C OpenGL Graphics Math (CGLM), OpenGL Graphics Math (GLM), Industrial Light & Magic Base (ILMBase), DirectX Math, and Unreal Engine 4.
TL;DR: How Realtime Math stands out
I believe Realtime Math stands out for a few reasons.
It is geared for high performance, deeply hot code. Most functions will end up inlined but the price to pay is an API that is a bit more verbose as a result of being C-style. When the need arises to use intrinsics, it gets out of the way and lets you do your thing. Only two libraries had what I would call optimal inlinability: Realtime Math and DirectX Math. Only those two libraries properly support the __vectorcall calling convention explicitly and only RTM handles GCC and Clang argument passing explicitly.
While it still needs a bit of love, quaternions are a first class citizen and it is the only standalone open source library I could find that supports QVV transforms (a rotation quaternion, a 3d scale vector, and a translation vector).
Realtime Math uses a coding style similar to the C++ standard library and feels clean and natural to read and write.
It consists entirely of C++11 headers, it runs almost everywhere, it supports 64 bit floating point arithmetic, and it sports a very permissive MIT license.
License
ACL is open source and uses the MIT license. I am never keen on adding dependencies and if I really have to, I want a permissive license free of constraints.
Library |
License |
Realtime Math |
MIT |
MathFu |
Apache 2.0 |
vectorial |
BSD 2-clause |
VectorialPlusPlus |
BSD 2-clause |
CGLM |
MIT |
GLM |
Modified MIT |
ILMBase |
Custom but permissive |
DirectX Math |
MIT |
Unreal Engine 4 |
UE4 EULA |
For simplicity and ease of integration, I want ACL to be entirely made of C++11 headers. This also constrains any dependencies to the same requirement.
Library |
Header Only |
Realtime Math |
Yes |
MathFu |
Yes |
vectorial |
Yes |
VectorialPlusPlus |
Yes |
CGLM |
Yes (optional lib) |
GLM |
Yes |
ILMBase |
No |
DirectX Math |
Yes |
Unreal Engine 4 |
No |
Verbosity, readability, and power
An important requirement for a math library is to be reasonably concise with average code without getting in the way if the need arises to dive right into raw intrinsics. In my experience, general math type abstractions take you very far but in order to squeeze out every cycle it is sometimes necessary to write custom per platform code. When this is required, it is important for the library to not hide its internals and leave the door open.
I am personally more a fan of C-style interfaces for a math library for various reasons: I can infer very well what happens under the hood (I have seen many libraries make fancy use of some operators that leave many newcomers to wonder what they do) and they are optimal for performance as we will discuss later. The downside of course is that they tend to be a bit more verbose. However, this largely boils down to a matter of personal taste.
vectorial is one of the few libraries that offers both a C-style interface and C++ wrappers and at the other end of the spectrum DirectX Math has both a namespace and a prefix for every type, constant and function.
Library |
Verbosity |
Realtime Math |
Medium (C-style) |
MathFu |
Light (C++ wrappers) |
vectorial |
Light (C++ wrappers) and Medium (C-style) |
VectorialPlusPlus |
Light (C++ wrappers) |
CGLM |
Medium (C-style) |
GLM |
Light (C++ wrappers) |
ILMBase |
Light (C++ wrappers) |
DirectX Math |
Medium++ (C-style with prefix and namespace) |
Unreal Engine 4 |
Light (C++ wrappers) |
It is very common for C-style math APIs to typedef their types to the underlying SIMD type. Realtime Math, DirectX Math, and many others do this. While this is great for performance, it does raise one problem: type safety is reduced. While usually those interfaces will opt to not expose proper vector2 or vector3 types and instead rely on functions that simply ignore the extra components, it doesn’t work so well when vector4 and quaternions are mixed. Only Realtime Math, DirectX Math and CGLM have quaternions with C-style interfaces but only the first two have a distinct type for quaternions when SIMD intrinsics are disabled. This somewhat mitigates the issue because with both Realtime Math and DirectX Math you can compile without intrinsics and still have type safety validated there. Although at the end of the day, all three have functions with distinct prefixes for vector and quaternion math and as such type safety is unlikely to be an issue.
Type and feature support
By virtue or being an animation compression library, ACL’s needs are a bit different from a traditional realtime application. This dictated the need I had for specific types and features. I had no need for general 3x3 or 4x4 matrices as well as 2D vectors which are more commonly used in gameplay and rendering. However, 3x4 affine matrices, 3D and 4D vectors, quaternions, and QVV transforms (a quaternion, a vector3 translation, and a vector3 scale) are of critical importance. Those types are front and center in an animation runtime and I needed them to be fully featured and fast. Most of the libraries under review had way more features than I cared for (mostly for rendering) but generally missed proper or any support for quaternions and QVV transforms.
MathFu appears to have a bug where the Matrix 4x4 SIMD template specialization isn’t included by default and its quaternions are 32 bytes instead of the ideal 16 due to alignment constraints.
VectorialPlusPlus quaternions also take 32 bytes instead of 16 due to alignment constraints and most of their quaternion code appears to be scalar.
UE 4 is notable for being the only other library to support QVV and it does offer a VectorRegister type to support SIMD for Vector2/3/4 although most of the code written in the engine uses the scalar version.
Library |
Vector2 |
Vector3 |
Vector4 |
Quaternion |
Matrix 3x3 |
Matrix 4x4 |
Matrix 3x4 |
QVV |
Realtime Math |
|
SIMD |
SIMD |
SIMD |
SIMD |
SIMD |
SIMD |
SIMD |
MathFu |
SIMD |
SIMD |
SIMD |
Partial SIMD |
Scalar |
SIMD |
Scalar |
|
vectorial |
|
SIMD |
SIMD |
|
|
SIMD |
|
|
VectorialPlusPlus |
SIMD |
SIMD |
SIMD |
Scalar |
SIMD |
SIMD |
|
|
CGLM |
|
SIMD |
SIMD |
SIMD |
SIMD |
SIMD |
SIMD |
|
GLM |
SIMD |
SIMD |
SIMD |
Partial SIMD |
SIMD |
SIMD |
SIMD |
|
ILMBase |
Scalar |
Scalar |
Scalar |
Scalar |
Scalar |
Scalar |
|
|
DirectX Math |
SIMD |
SIMD |
SIMD |
SIMD |
|
SIMD |
|
|
Unreal Engine 4 |
Scalar |
Scalar |
Scalar |
SIMD |
|
SIMD |
|
SIMD |
SIMD architecture support
Equally important was the SIMD architecture support. I want to run ACL everywhere with the best performance possible, especially on mobile. SSE, AVX, and NEON are all equally important to me.
Worth noting that 2 years ago DirectX NEON support appeared almost exclusively to be for Windows ARM NEON and I have no idea if it runs on iOS or Android even today.
Library |
SSE |
AVX |
NEON |
Realtime Math |
Yes |
Yes |
Yes |
MathFu |
Yes |
|
Yes |
vectorial |
Yes |
|
Yes |
VectorialPlusPlus |
Yes |
|
Partial |
CGLM |
Yes |
Yes |
Partial |
GLM |
Yes |
|
|
ILMBase |
|
|
|
DirectX Math |
Yes |
Yes |
Yes |
Unreal Engine 4 |
Yes |
Yes |
Yes |
Here things are a bit more complicated as libraries will list platforms but not compilers or compilers but not platforms. I need ACL to run everywhere and this means limiting myself to C++11 features.
- Realtime Math: Windows (VS2015, VS2017) x86 and x64, Linux (gcc5, gcc6, gcc7, gcc8, clang4, clang5, clang6) x86 and x64, OS X (Xcode 8.3, Xcode 9.4, Xcode 10.1) x86 and x64, Android clang ARMv7-A and ARM64, iOS (Xcode 8.3, Xcode 9.4, Xcode 10.1) ARM64
- MathFu: Windows, Linux, OS X, Android
- vectorial: Unlisted but probably Windows, Linux, OS X, Android, and iOS
- VectorialPlusPlus: Unlisted but probably Windows
- CGLM: Windows, Unix, and probably everywhere
- GLM: VS2013+, Apple Clang 6, GCC 4.7+, ICC XE 2013+, LLVM 3.4+, CUDA 7+
- ILMBase: Unlisted but probably Windows, Linux, OS X
- DirectX Math: VS2015 and VS2017, possibly elsewhere
- Unreal Engine 4: Windows (VS2015, VS2017) x64, Linux x64, OS X x64, Android ARMv7-A (no NEON) and ARM64, iOS ARM64
Continuous integration support
Continuous integration is a critical part of modern software development especially with C++ when multiple platforms are supported and maintained.
Library |
Continuous Integration |
Realtime Math |
Yes |
MathFu |
No |
vectorial |
No |
VectorialPlusPlus |
No |
CGLM |
Yes |
GLM |
Yes |
ILMBase |
No |
DirectX Math |
No |
Unreal Engine 4 |
Not public |
Dependencies
I’m not personally a big fan of pulling in tons of dependencies, especially for a math library. As mentioned earlier, the Unreal Engine 4 math library isn’t really usable on its own because of this but is included regardless.
Library |
Dependencies |
Realtime Math |
|
MathFu |
vectorial (BSD 2-clause) |
vectorial |
|
VectorialPlusPlus |
HandyCPP (custom license) |
CGLM |
|
GLM |
|
ILMBase |
|
DirectX Math |
|
Unreal Engine 4 |
Unreal Engine 4 |
Floating point support
When I got started with ACL, I wasn’t sure at the time if 64 bit floating point arithmetic might offer superior accuracy or not and if it would be worth using. As a result, I needed the math code to support both float32 and float64 types for everything with a seamless API between the two for quick testing. It later turned out that the extra floating point precision isn’t helping enough to be worth using.
Library |
Float 32 Support |
Float 64 Support |
Realtime Math |
Yes |
Yes (partial SIMD) |
MathFu |
Yes |
Yes (no SIMD) |
vectorial |
Yes |
|
VectorialPlusPlus |
Yes |
Yes (partial SIMD) |
CGLM |
Yes |
|
GLM |
Yes |
|
ILMBase |
Yes (no SIMD) |
Yes (no SIMD) |
DirectX Math |
Yes |
|
Unreal Engine 4 |
Yes |
|
Inlinability
Due to the critical need for ACL to be as fast as possible on every platform, having the bulk of the math operations be inline is very important. Many things impact whether a function is inlined by the compiler but two stand out:
- Simple and short functions inline better
- Passing arguments by register needs fewer instructions which inlines better
Thankfully, most math function are fairly simple and short: add, mul, div, etc. C-style functions will generally have a slight advantage over C++ wrappers mainly because they also must track the implicit this pointer being passed around even if ultimately it is optimized out inside the caller. When the compiler needs to determine if it can inline a function, it uses a heuristic and the size of the intermediate assembly/IR/AST most likely plays a role. Generally speaking, C++ wrapper functions that are short will inline just fine but some operations have a harder time due to their size: matrix 4x4 multiplication, quaternion multiplication, and quaternion interpolation. For this reason, I personally favor a C-style API for this sort of code.
The second point is not to be underestimated. Most of the libraries in the list either take the arguments by value or by const reference. While passing SIMD types by value does the right thing on ARM and passes them by register (up to 4), it does not work for aggregate types like matrices and it does not work with the default x64 calling convention with MSVC. In order to be able to pass SIMD types by register with MSVC, you must use its __vectorcall calling convention. It also works for aggregate and wrapper types. Up to 6 registers can be used for this. On desktop and Xbox One, using __vectorcall is critical for high performance code and sadly, most libraries do not support it explicitly (and not all support it implicitly if the whole compilation unit is forced to use that calling convention). With Visual Studio 2015, __vectorcall is the difference between having quaternion interpolation getting inlined or not. When I added support for it in ACL, I measured a roughly 5% speedup during the decompression.
Note that once a function is inlined, whether the arguments are passed by register or not typically does not impact the generated assembly although it sometimes does (at least with MSVC especially when AVX is enabled).
Some libraries which use a generic vector template class with specializations for SIMD (like MathFu) sometime end up passing *float32 arguments by const-reference instead of by value which is often suboptimal when not inlined.*
Library |
Inlinability |
Register Passing |
Realtime Math |
Optimal (C-style + by register) |
Explicit (everywhere) |
MathFu |
Decent (C++ wrappers) |
None |
vectorial |
Good (C-style), Decent (C++ wrappers) |
Implicit (C-style and ARM only) |
VectorialPlusPlus |
Decent (C++ wrappers) |
None |
CGLM |
Good (C-style) |
None |
GLM |
Decent (C++ wrappers) |
None |
ILMBase |
Decent (C++ wrappers) |
None |
DirectX Math |
Optimal (C-style + by register) |
Explicit (vectorcall and ARM only) |
Unreal Engine 4 |
Decent (C++ wrappers) |
None |
Multiplication order
An important point of contention is how things are multiplied. As the list below shows, the OpenGL way is by far the most popular for open source math libraries.
It all boils down to whether vectors are represented as a row or as a column. In the former case, multiplication with a matrix takes the form v' = vM
while in the later case we have v' = Mv
. Linear algebra typically treats vectors as columns and OpenGL opted to use that convention for that reason. If you think of matrices as functions that modify an input and return an output it ends up reading like this: result = object_to_world(local_to_object(input))
. This reads right-to-left as is common with nested function evaluation. In my opinion, this is quite awkward to work with as most modern programming languages (and western languages) read left-to-right. Most linear algebra formulas use abstract letters and names for things which somewhat hides this nuance but when I write code, I try to keep my matrix names as clear as possible: what space are the input and output in. While you could technically reverse the naming result = world_from_object * object_from_local * input
so it at least reads decently right-to-left, it’s still harder to reason with because just about everything we work with in the world goes from somewhere to somewhere else and not the other way around: trains, buses, planes, Monday to Friday, 5@7, etc.
On the other hand, DirectX uses row vectors and ends up with the much more natural: result = input * local_to_object * object_to_world
. Your input is in local space, it gets transformed into object space before finally ending up in world space. Clean, clear, and readable. If you instead multiply the two matrices together on their own, you get the clear local_to_world = local_to_object * object_to_world
instead of the awkward local_to_world = object_to_world * local_to_object
you would get with OpenGL and column vectors.
At the end of the day, which way you choose largely boils down to a personal choice (or whatever library you use for rendering) as I don’t think there’s a big performance difference between the two on modern hardware. For ACL, all its output data is in local space and although we evaluate the error in world space internally, this is entirely transparent to the client application and it is free to use either convention.
Library |
Multiplication Style |
Realtime Math |
DirectX |
MathFu |
OpenGL |
vectorial |
OpenGL |
VectorialPlusPlus |
OpenGL |
CGLM |
OpenGL |
GLM |
OpenGL |
ILMBase |
OpenGL |
DirectX Math |
DirectX |
Unreal Engine 4 |
DirectX |
Conclusion
Ultimately, which math library you choose for a particular project boils down to a matter of personal preference to a large extent. For the vast majority of the code you’ll write, the performance and code generation is likely to be very close if not identical. Two years ago, I knew regardless of which option I picked I would have to do a lot of work to add what was missing. This greatly motivated me to just start from scratch as many middleware do and I do not regret the experience or results.
My top two favorite libraries are Realtime Math and DirectX Math. Both are quite similar today although DirectX Math wasn’t quite as attractive when I started.
Next steps
Over the next few days I will populate various issues on GitHub to document things that are missing or that could benefit from some love.
A core part that is partially missing at the moment is the quantization and packing logic that ACL already contains. I have not migrated that code yet in large part because I am not sure how to best expose it in a clean and consistent API. I do believe it belongs in RTM where everyone can benefit from it.
ACL does not yet use RTM but that migration is planned for ACL v2.0.
08 Sep 2018
I am excited to announce the open source Animation Compression Library has reached v1.1 along with an updated Unreal Engine 4 plugin v0.2.
ACL now beats Unreal Engine 4 on all the important metrics. The plugin is 1.7x smaller, 3x more accurate, 2.5x faster to compress, and up to 4.8x faster to decompress!
What’s new
The latest release focused on decompression performance. ACL v1.1 is about 30% faster than the previous version on every platform when decompressing a whole pose. Decompressing a single bone, a common operation in Unreal, is now about 3-4x faster. Also, ARM-based products will now use NEON SIMD acceleration when available.
The UE4 plugin was reworked to be more tightly integrated and is about twice as fast compared to the previous version.
ACL has now reached a point where I can confidently say that it is the best overall animation compression algorithm in the video games industry. While other techniques might beat ACL on some of these metrics, beating it simultaneously on speed, size, and accuracy will prove to be very challenging. In particular, unlike other algorithms that offer very fast decompression, ACL has no extra runtime memory cost beyond the compressed clip data.
New data!
One year ago, Epic generously agreed to let me use the Paragon animations for research purposes. This helped me find and fix bugs in Unreal Engine and ACL, and see how well both animation compression approaches perform in a real game. Paragon also allows each release to be rigorously tested against a large, relevant, and varied data set.
I am excited to announce that Epic is allowing me to use Fortnite to further my research as well! While Paragon will continue to play its role in tracking compression performance and regression testing, Fortnite will allow me to measure decompression performance in real world scenarios much more easily. Testing with Fortnite should highlight new ways ACL can be improved further.
What’s next
I am shifting my focus to add animation compression plugin support to UE4 during the next few months. If everything goes well, when UE 4.22 is released next year, I will be able to add the ACL plugin to the Unreal Engine Marketplace for everyone to use, for free.
Proper plugin support will remove overhead and help make ACL’s in-game decompression faster still.
Due to the rigorous testing and extensive statistics extraction every release now requires, I expect the release cycle to slow down. I will aim to perform non-bug fix releases about twice a year.
Here is a quick glance of how well it performs on the animations from Paragon:
|
ACL Plugin v0.2.0 |
UE v4.19.2 |
Compressed size |
226.09 MB |
392.97 MB |
Compression ratio |
18.91 : 1 |
10.88 : 1 |
Compression time |
6h 4m 18.21s |
15h 10m 23.56s |
Bone Error 99th percentile |
0.0116 cm |
0.0328 cm |
Samples below 0.01 cm error threshold |
98.85 % |
84.88 % |
The 99th percentile and the number of samples below the 0.01 cm error threshold are calculated by measuring the world-space error of every bone at every sample in each of the 6558 animation clips. To put this into perspective, over 99 % of the compressed data has an error lower than the width of a human hair. More details on how the error is measured can be found here.
Decompression performance is currently tracked with the Matinee fight scene. The troopers have around 70 bones each while the main trooper has 541.
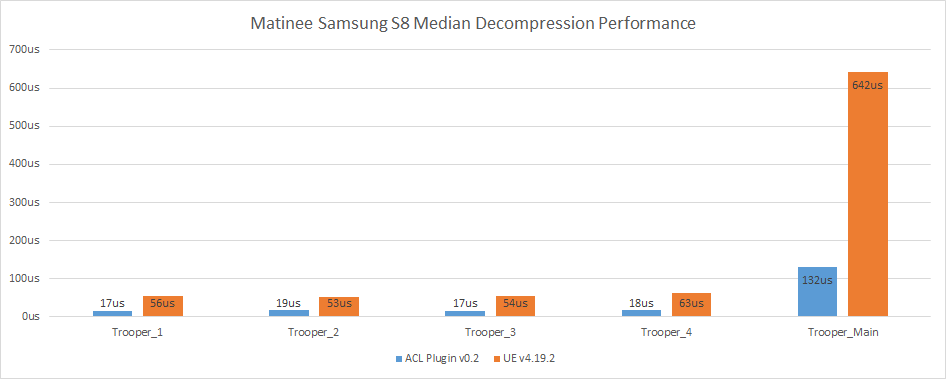
Much care was taken to ensure that ACL has consistent decompression performance. The following two images show the time taken to decompress a pose at every point of the Matinee fight scene which highlights how regular ACL is.
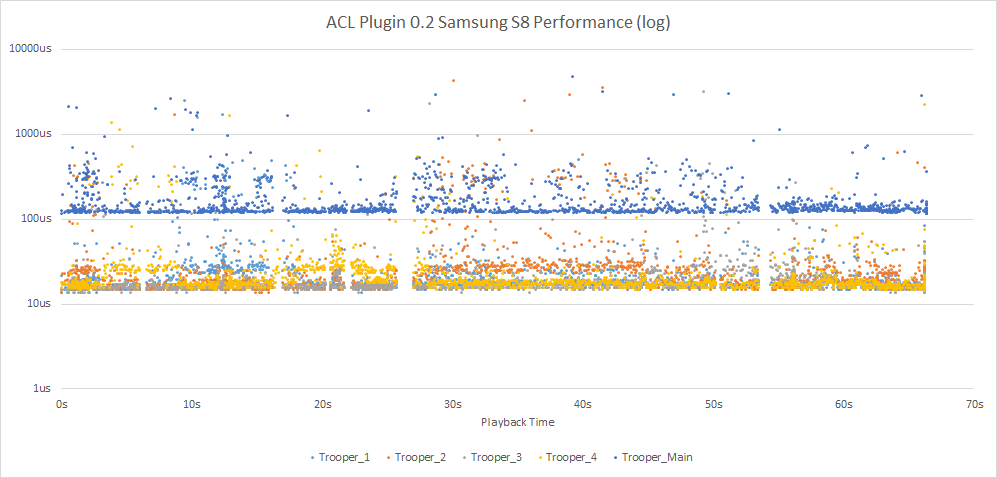
It also has consistent decompression performance regardless of the playback direction and it works on every modern platform making it a safe choice when using it as the default algorithm in your games.
Overall, ACL is ideal for games with large amounts of animations playing concurrently such as those with large crowds, MMOs, and e-sports as well as those that run on mobile or slower platforms.
21 Jul 2018
The long awaited ACL v1.0 release is finally here! And it comes with the brand new Unreal Engine 4 plugin v0.1! It took over 15 months of late nights, days off, and weekends to reach this point and I couldn’t be more pleased with the results.
Recap
The core idea behind ACL was to explore a different way to perform animation compression, one that departed from classic methods. Unlike the vast majority of algorithms in the wild, it uses bit aligned values as opposed to naturally aligned integers. This is slower to unpack but I hoped to compensate by not performing any sort of key reduction. By retaining every sample, the data is uniform in memory and offsets are trivially calculated, keeping things fast, the memory touched contiguous, and the hardware happy. While the technique itself isn’t novel and is often used with compression algorithms in other fields, to my knowledge it had never been tried to the extent ACL pushes it with animation compression, at least not publicly.
Very early, the technique proved competitive and over time it emerged as a superior alternative over traditional techniques involving key reduction. I then spent about 8 months writing the necessary infrastructure to make ACL not only production ready but production quality: unit tests were written, extensive regression tests were introduced, documentation was added as well as comments, scripts to replicate the results, cross platform support (ACL now runs on every platform!), etc. All that good stuff that one would expect from a professional product.
But don’t take my word for it! Check out the 100% C++ code (MIT license), the statistics below, and take the plugin out for a spin!
While ACL provides various synthetic test hardnesses to benchmark and extract statistics, nothing beats running it within a real game engine. This is where the UE4 plugin comes in and really shines. Just as with ACL, three data sets are measured: CMU, Paragon, and the Matinee fight scene.
Note that there are small differences between measuring with the UE4 plugin and with the ACL test harnesses due to implementation choices in the plugin.
Carnegie-Mellon University (CMU)
|
ACL Plugin v0.1.0 |
UE v4.19.2 |
Compressed size |
70.60 MB |
99.94 MB |
Compression ratio |
20.25 : 1 |
14.30 : 1 |
Max error |
0.0722 cm |
0.0996 cm |
Compression time |
34m 30.51s |
1h 27m 40.15s |
ACL was smaller for 2532 clips (99.92 %)
ACL was more accurate for 2486 clips (98.11 %)
ACL has faster compression for 2534 clips (100.00 %)
ACL was smaller, better, and faster for 2484 clips (98.03 %)
Would the ACL Plugin have been included in the Automatic Compression permutations tried, it would have won for 2534 clips (100.00 %)
Data tracked here by the plugin, and here by ACL.
Paragon
|
ACL Plugin v0.1.0 |
UE v4.19.2 |
Compressed size |
226.02 MB |
392.97 MB |
Compression ratio |
18.92 : 1 |
10.88 : 1 |
Max error |
0.8566 cm |
0.6424 cm |
Compression time |
6h 35m 03.24s |
15h 10m 23.56s |
ACL was smaller for 6413 clips (97.79 %)
ACL was more accurate for 4972 clips (75.82 %)
ACL has faster compression for 5948 clips (90.70 %)
ACL was smaller, better, and faster for 4499 clips (68.60 %)
Would the ACL Plugin have been included in the Automatic Compression permutations tried, it would have won for 6098 clips (92.99 %)
Data tracked here by the plugin, and here by ACL.
Matinee fight scene
|
ACL Plugin v0.1.0 |
UE v4.19.2 |
Compressed size |
8.67 MB |
23.67 MB |
Compression ratio |
7.20 : 1 |
2.63 : 1 |
Max error |
0.0674 cm |
0.0672 cm |
Compression time |
52.44s |
54m 03.18s |
ACL was smaller for 1 clip (20 %)
ACL was more accurate for 4 clips (80 %)
ACL has faster compression for 5 clips (100 %)
ACL was smaller, better, and faster for 0 clip (0 %)
Would the ACL Plugin have been included in the Automatic Compression permutations tried, it would have won for 3 clips (60 %)
Data tracked here by the plugin, and here by ACL.
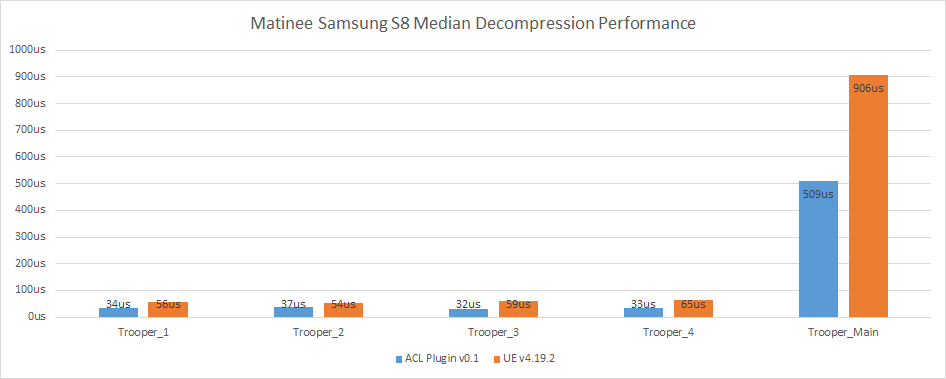
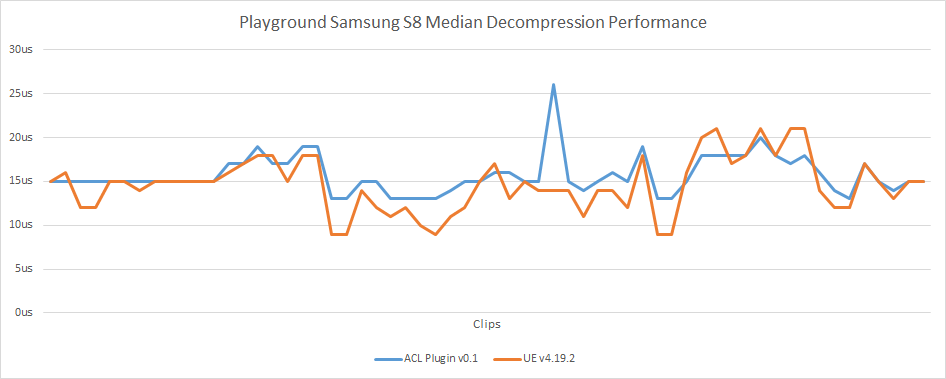
Data tracked here by the plugin, and here by ACL (they also include other platforms and more data).
As the numbers clearly show, ACL beats UE4 across every compression metric, sometimes by a significant margin: it is MUCH faster to compress, the quality is just as good, and the memory footprint is significantly reduced. ACL achieves all of this with default settings that animators rarely if ever need to tweak. What’s not to love?
However, the ACL decompression performance is sometimes ahead, sometimes behind, or the same. There are a few reasons for this, most of which I am hoping to fix in the next version to take the lead: NEON (SIMD) is not yet used on ARM, the ACL plugin needlessly performs MUCH more work than UE4 when decompressing, and many low hanging fruits were left to be fixed post-1.0 release.
ACL is just getting started!
How to use the ACL Plugin
As the documentation states here, a few minor engine changes are required in order to support the ACL plugin. These changes mostly consist of bug fixes and changes to expose the necessary hooks to plugins.
For the time being, the plugin is not yet on the marketplace as it is not fully plug-and-play. However, this summer I am working with Epic to introduce the necessary changes in order to publish the ACL plugin on the marketplace. Stay tuned!
Note that the ACL Plugin will reach v1.0 once it can be published on the marketplace but it is production ready regardless.
What’s new in ACL v1.0
Few things actually changed in between v0.8 and v1.0. Most of the changes revolved around minor additions, documentation updates, etc. There are two notable changes:
- The first is visible in the decompression graphs: we now yield the thread before measuring every sample. This helps ensure more stable results by reducing the likelihood that the kernel will swap out the thread and interrupt it while executing the decompression code.
- The second is visible in the compression stats for Paragon: a bug was causing the visible error to sometimes be partially hidden when 3D scale is present. While the new version is not less accurate than the previous, the measured error can be higher in very rare cases (only 1 clip is higher).
Regardless, the measuring should now be much more stable.
What’s next
The next release of ACL will focus on improving the compression and decompression performance. While ACL was built from the ground up to be fast to decompress; so far the focus has been on making sure things function properly and safely to establish a solid baseline to work with. Now that this work is done, the fun part can begin: making it the best it can be! I have many improvements planned and while some of them will make it in v1.1, others will have to wait for future versions.
Special care will be taken to make sure ACL performs at its best in UE4 but there is no reason why it couldn’t be used in your own favorite game engine or animation middleware. Developing with UE4 is easier for me in large part because of my past experience with it, my relationship with Epic, and the fact that it is open source. Other game engines like Unity explicitly forbid their use for benchmarking purposes in their EULA which prevents me from publishing any results without prior written agreement form their legal departement. Furthermore, without access to the source code, creating a plugin for it requires a lot more work. In due time, I hope to support Unity, Godot, and anyone else willing to try it out.